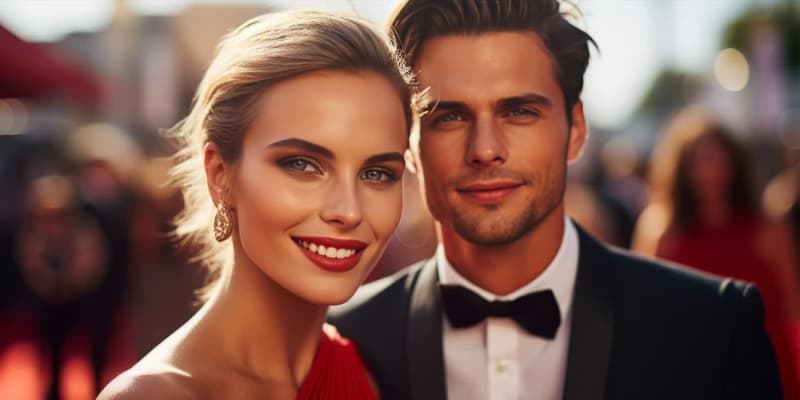
A study utilizing machine learning and various statistical techniques on a large dataset of celebrity photographs found no link between the facial similarity of celebrity couples and the duration of their relationships. The researchers discovered that only a very weak prediction of relationship longevity could be made based on the properties of the entire face. The paper was published in Symmetry.
Facial attractiveness plays a significant role in shaping social perceptions and interactions. Attractive individuals are generally perceived more positively than less attractive ones. They generally tend to receive favorable treatment and judgments in various social contexts including employment, romantic relationships, and social networking. This bias towards attractiveness can lead to advantages in personal and professional life, but it also perpetuates societal standards and pressures related to physical appearance.
Studies have shown that attractive facial features trigger specific responses in the areas of the brain linked with rewards (such as the orbitofrontal cortex and the amygdala). For the human brain, faces of other people are one of the most important types of stimuli. As soon as a face is seen, different parts of the human brain start working together to recognize it and respond to it. This allow individuals to identify faces and assess traits like trustworthiness and aggressiveness in less than a one tenth of a second. But can facial features be used to predict how long one’s romantic relationship will last?
Study author Veronika Shavlokhova and her colleagues wanted to find out. They noted that many studies on romantic relationships emphasize facial symmetry as a factor in partner selection and marital satisfaction. Other studies propose that similarity between romantic partners is an important factor determining the course of the relationship. With this in mind, these researchers conducted a study aiming to explore whether dissimilarity of faces of individuals in a couple could be used to predict the duration of their romantic relationship.
They used machine learning techniques to analyze the CELEB-A dataset, a collection of 202,599 facial images of 10,177 celebrities taken from the web and maintained by the Multimedia Lab at the Chinese University of Hong Kong. Images included in this collection came from a diverse group of countries and regions across the globe.
For their analysis, the researchers selected one image per celebrity and searched the internet to identify those in romantic relationships or marriages with one another, along with the duration of these relationships. This information was included in the analysis wherever the duration could be clearly established. Ultimately, they compiled a dataset of 1,822 celebrity pairs, complete with images and information on the length and nature of their relationships.
They used DenseNet machine learning architecture for their analysis. DenseNet or Densely Connected Convolutional Network is a type of deep convolutional neural networks that features dense connections between layers, where each layer receives inputs from all preceding layers, facilitating feature reuse and reducing the number of parameters.
In general, deep convolutional networks or DCNs are a class of artificial neural networks that are particularly well-suited for processing data with a grid-like structure, such as images. They consist of multiple layers of filters that automatically learn to extract relevant features from the input data. In face recognition, DCNs can be trained to identify and distinguish between different individuals’ faces by learning complex patterns and characteristics specific to each person’s facial features.
Neural networks are a class of algorithms inspired by the structure and function of the human brain. They are widely used to model complex patterns and relationships in data in order to perform tasks such as classification, prediction, or pattern recognition. In their analysis, the study authors used the DenseNet DCN to compare faces of celebrities within the pairs they identified.
Results showed that the average duration of a relationship between celebrities was 109 months. However, the median was only 48 months. The dissimilarity of faces of celebrities within a pair varied to different degrees across various facial regions. They tended to be the highest for the face as a whole followed by the dissimilarity of eyes, nose, and the mouth regions (in that order).
However, these dissimilarities were not associated with the duration of a couple’s relationship. There were also no differences in average facial dissimilarity levels in married couples and partners who were not married. On an unrelated note, partners who had higher levels of dissimilarity of the whole face were somewhat more likely to have dissimilar left eyes.
Further analysis enabled the researchers to develop a statistical model that offered a very weak prediction of relationship duration based on whole facial properties. The accuracy of this prediction was only very slightly better than random guessing.
“Our study highlights the intricacies involved in predicting relationship outcomes solely based on facial attributes, as the results do not provide substantial evidence to support a direct correlation between facial dissimilarity and partnership duration. This underscores the intricate nature of partnership dynamics, suggesting that other nuanced factors may play a more crucial role in determining the course of relationships,” the study authors concluded.
The study makes a pioneering approach in using AI-based image analysis for exploring relationships. However, it should be noted that the analyses were made on a selected group of images, with only one image representing each person. It is possible that using a wider sample of images could produce different results. Additionally, the study was conducted on images of celebrities, a group that might not represent the general population well. Results might not be the same if a similar analysis was conducted on individuals more representative of the general population.
The paper, “Assessing the Role of Facial Symmetry and Asymmetry between Partners in Predicting Relationship Duration: A Pilot Deep Learning Analysis of Celebrity Couples”, was authored by Veronika Shavlokhova, Andreas Vollmer, Christian Stoll, Michael Vollmer, Gernot Michael Lang, and Babak Sarav.