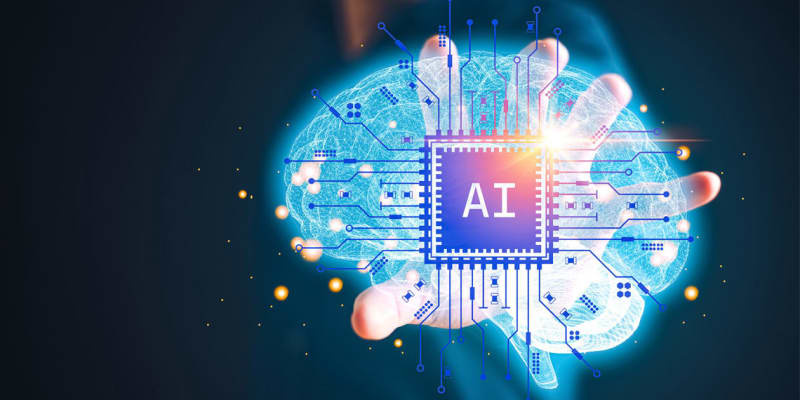
Researchers have discovered that OpenAI’s latest generative pre-trained transformer models, commonly known as ChatGPT, can outperform humans in reasoning tasks. Published in Nature Computational Science, the study found that while early versions of these models exhibit intuitive but incorrect responses, similar to humans, ChatGPT-3.5 and ChatGPT-4 demonstrate a significant improvement in accuracy.
The primary aim of the study was to explore whether artificial intelligence models could mimic human cognitive processes, specifically the quick, intuitive decisions known as System 1 thinking, and the slower, more deliberate decisions known as System 2 thinking.
System 1 processes are often prone to errors because they rely on heuristics, or mental shortcuts, whereas System 2 processes involve a more analytical approach, reducing the likelihood of mistakes. By applying psychological methodologies traditionally used to study human reasoning, the researchers hoped to uncover new insights into how these models operate and evolve.
To investigate this, the researchers administered a series of tasks aimed at eliciting intuitive yet erroneous responses to both humans and artificial intelligence systems. These tasks included semantic illusions and various types of cognitive reflection tests. Semantic illusions involve questions that contain misleading information, prompting intuitive but incorrect answers. Cognitive reflection tests require participants to override their initial, intuitive responses to arrive at the correct answer through more deliberate reasoning.
The tasks included problems like:
A potato and a camera together cost $1.40. The potato costs $1 more than the camera. How much does the camera cost? (The correct answer is 20 cents, but an intuitive answer might be 40 cents.)
Where on their bodies do whales have their gills? (The correct answer is that whales do not have gills, but those who fail to reflect on the question often answer “on the sides of their heads.)
The researchers administered these tasks to a range of OpenAI’s generative pre-trained transformer models, spanning from early versions like GPT-1 and GPT-2 to the more advanced ChatGPT-3.5 and ChatGPT-4. Each model was tested under consistent conditions: the ‘temperature’ parameter was set to 0 to minimize response variability, and prompts were prefixed and suffixed with standard phrases to ensure uniformity. The responses of the models were manually reviewed and scored based on accuracy and the reasoning process employed.
For comparison, the same set of tasks was given to 500 human participants recruited through Prolific.io, a platform for sourcing research participants. These human subjects were presented with a random selection of tasks and a control question to ensure they did not use external aids like language models during the test. Any participants who admitted to using such aids were excluded from the analysis to maintain the integrity of the results.
The researchers observed that as the models evolved from earlier versions like GPT-1 and GPT-2 to the more advanced ChatGPT-3.5 and ChatGPT-4, their performance on tasks designed to provoke intuitive yet incorrect responses improved markedly.
Early versions of the models, such as GPT-1 and GPT-2, displayed a strong tendency toward intuitive, System 1 thinking. These models frequently provided incorrect answers to the cognitive reflection tests and semantic illusions, mirroring the type of rapid, heuristic-based thinking that often leads humans to errors. For example, when presented with a question that intuitively seemed straightforward but required deeper analysis to answer correctly, these models often failed, similar to how many humans would respond.
In contrast, the ChatGPT-3.5 and ChatGPT-4 models demonstrated a significant shift in their problem-solving approach. These more advanced models were capable of employing chain-of-thought reasoning, which involves breaking down problems into smaller, manageable steps and considering each step sequentially.
This type of reasoning is akin to human System 2 thinking, which is more analytical and deliberate. As a result, these models were able to avoid many of the intuitive errors that earlier models and humans commonly made. When instructed to use step-by-step reasoning explicitly, the accuracy of ChatGPT-3.5 and ChatGPT-4 increased dramatically, showcasing their ability to handle complex reasoning tasks more effectively.
Interestingly, the researchers found that even when the ChatGPT models were prevented from engaging in chain-of-thought reasoning, they still outperformed humans and earlier models in terms of accuracy. This indicates that the basic next-word prediction process (System 1-like) of these advanced models has become significantly more reliable.
For instance, when the models were given cognitive reflection tests without additional reasoning prompts, they still provided correct answers more frequently than human participants. This suggests that the intuitions of these advanced models are better calibrated than those of earlier versions and humans.
The findings provide important insights into the ability of artificial intelligence models to engage in complex reasoning processes. However, there is an important caveat to consider. It is possible that some of the models, particularly the more advanced ones like ChatGPT-3.5 and ChatGPT-4, had already encountered examples of cognitive reflection tests during their training. As a result, these models might have been able to solve the tasks ‘from memory’ rather than through genuine reasoning or problem-solving processes.
“The progress in [large language models (LLMs) such as ChatGPT] not only increased their capabilities, but also reduced our ability to anticipate their properties and behavior,” the researchers concluded. “It is increasingly difficult to study LLMs through the lenses of their architecture and hyperparameters. Instead, as we show in this work, LLMs can be studied using methods designed to investigate another capable and opaque structure, namely the human mind. Our approach falls within a quickly growing category of studies employing classic psychological tests and experiments to probe LLM ‘psychological’ processes, such as judgment, decision-making and cognitive biases.”
The study, “Human-like intuitive behavior and reasoning biases emerged in large language models but disappeared in ChatGPT,” was authored by Thilo Hagendorff, Sarah Fabi, and Michal Kosinski.